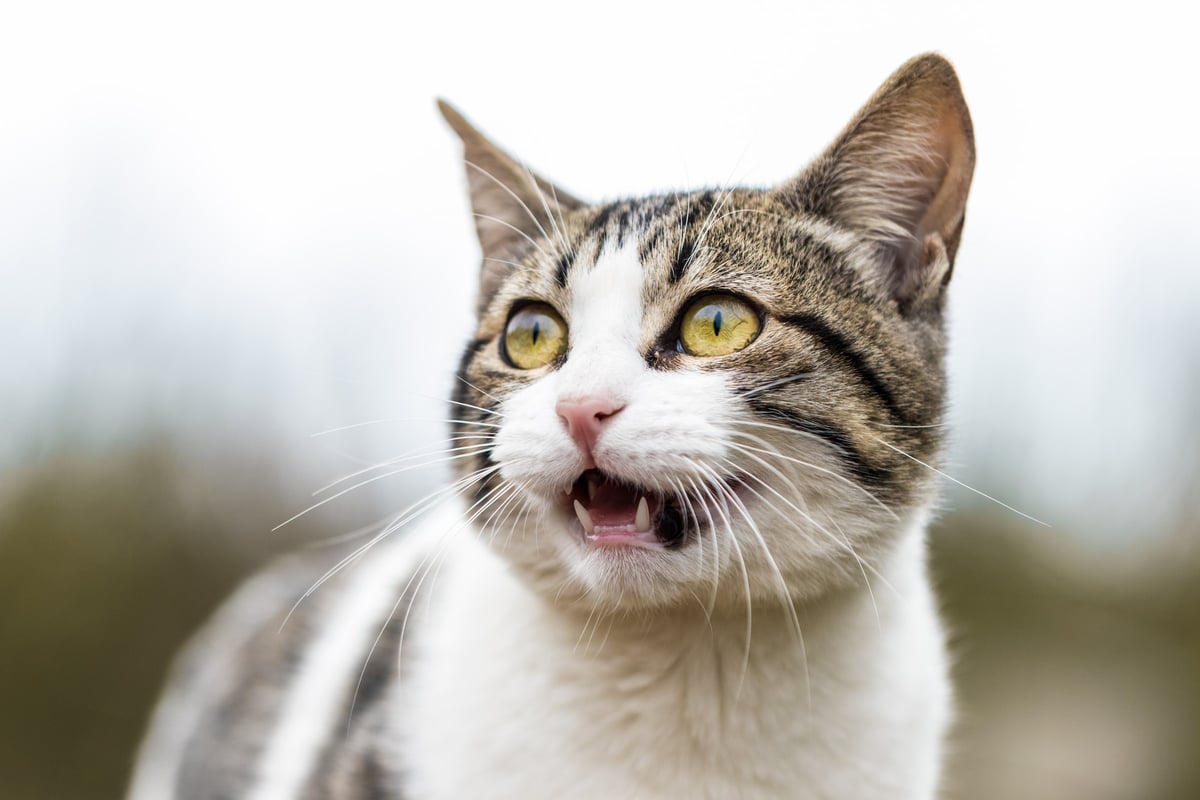
This article isn't focused on a specific role but on obstacles you may encounter in your day-to-day work. It touches on building trust between the Business and Data Science, securing leadership support, identifying opportunities in data and AI, advocating for infrastructure & research projects, emphasizing value-centric outcomes, underscoring the importance of data & AI strategies and teamwork in Data Science. While the issues described in the following paragraphs can certainly overlap, the aim is to highlight different aspects of related problems.
📉 You experience a lack of leadership support or excessive bureaucratic obstacles
Your reporting structure might be far from perfect. Data teams can be scattered throughout the organization, sometimes led by people who don't have any background in data and AI. No matter how frequently they emphasize the importance of data to support the hype, this doesn't necessarily translate into approval for significant initiatives. Not to say about initiatives that extend beyond the data team. They often wither due to miscommunication or resistance from other departments. Your manager might not even fully understand your role and responsibilities.
🔮 Business expects you to transform data chaos into game-changing opportunities, leaving them uninvolved
Putting engineers in charge of opportunity discovery allows technology to drive the process. This approach is expensive and ineffective, as we need a connection to the Business, not build a thing. Otherwise, there's a risk of developing some appealing functionality in search of a use case, or, in other words - that no one needs. Picture a scenario where you start by going through terabytes of data to pinpoint potential opportunities - this is a gamble at best. Sometimes, it might feel like a fishing expedition or, worse, like scavenging through a dumpster. If data is gathered and curated intentionally, starting from a problem, even a small amount of data can be enough to power a product. Alternatively, beginning with a solution in mind can steer us even further from tangible outcomes. In this era, feasibility isn't usually the primary issue. It's only a part of the larger puzzle. Not every problem should be solved with data and AI. Even a mighty LLM is not a silver bullet, no matter the size of your data warehouse. Yet there are things that data and AI handle better than anything else. Tasks such as planning, prioritizing, and final decision-making are all leadership challenges, so they must be involved. Someone needs to provide the leadership with frameworks and set up processes that allow ideas to flow from every corner of the Business. Someone needs to become a funnel for the ideas, educate others about data and AI, and keep everyone engaged, so they bring higher-quality ideas next time. This way, the leadership will focus on their challenges - managing value-creation.
🏗️ Greenlighting data initiatives feels like moving mountains
Every time you pitch an infrastructure or research initiative focusing purely on technology, you reduce your chance of getting buy-in, regardless of how evident the need for implementation might seem to you. Alternatively, sometimes we solve a problem that doesn't truly exist from the Business's viewpoint. This can lead to us fooling ourselves into thinking we're making progress by creating expensive solutions that aren't really needed. You must ask, what will change once this initiative is delivered? Which metric will be affected? Is the company prepared for this? What opportunities will this unlock? What threats will it address? Will the outcomes justify the risks and costs of implementation? What is the ultimate value? Adopting a value-centric approach and utilizing communication frameworks can pave the way for gaining the required approvals.
🎢 There's an absence of a solid data or AI strategy, with ever-changing priorities
Data and AI present multiple paths a business could take, but not all of them make sense for the Business. Some companies try to explore these based on guesswork. Some follow the hype. Others stick to what's feasible today. None of these approaches are optimal. In fact, they increase the risk of being outpaced by competitors. It's the leadership's job to shape the core business strategy, but having a strategic partner in the tech world is also crucial. If no one advocates for data and AI - it will result in a high-cost missed opportunity. This partner can help extend the core strategy with technology strategies, focusing the limited resources of the entire Business on the most promising initiatives based on the technologies and articulated value. Often, there's something mistakenly labeled as a strategy, but these are typically tactics in disguise. The "what" and "how" are components of strategy implementation, not strategy itself. A genuine strategy focuses on the "why". It requires research of the business state, capabilities, competition, threats, and more. Due to low maturity, the Business may not even be ready to develop an AI strategy yet. However, your understanding of the technology landscape will be a significant asset. You can learn to present technology from a value-creation standpoint and translate the core strategy into technological terms. While this is a huge topic, you can follow practical tips to create levers for the Business as you gradually develop a strategic mindset.
🦄 Business sets unrealistic expectations for your role
Businesses sometimes hire professionals with a "we're not sure what we need, but we hope you do" mindset, expecting them to tackle all data-related tasks. This often results in a lose-lose situation: the Business doesn't reach its goals, and the professional ends up feeling frustrated. The effective application of Data Science needs a team approach. Each role is characterized by unique, high-value tasks that can't be automated. Every minute you spend on extra tasks is a minute not spent delivering unique value that no one else in the company can provide. A business shouldn't expect investors to buy into its narrative of leveraging data and AI without a dedicated team. Explaining the various roles and their value, tied to potential outcomes of the current research pipeline and roadmap, can help convince leadership to invest in additional hiring. As the team grows, work can be better distributed among different data roles, allowing each member to concentrate on what they do best.
Although I don't claim to have all the answers, we can dive into those areas and go beyond. This journey will be guided by the experience I've gained from my wins and setbacks, the practical frameworks I've put into practice, and the valuable insights I've gleaned from others. Don't hesitate to message me with your inquiries - I'm here for you.